Difference Between Algorithm and Artificial Intelligence
There have been a few false starts along the road to the “AI revolution”, and the term Machine Learning certainly gives marketers something new, shiny and, importantly, firmly grounded in the here-and-now, to offer. Machine Learning applications can read text and work out whether the person who wrote it is making a complaint or offering congratulations. They can also listen to a piece of music, decide whether it is likely to make someone happy or sad, and find other pieces of music to match the mood.
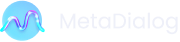
Machine Learning is a current application of AI based around the idea that we should really just be able to give machines access to data and let them learn for themselves. While the terms Data Science, Artificial Intelligence (AI), and Machine learning fall in the same domain and are connected, they have specific applications and meanings. There may be overlaps in these domains now and then, but each of these three terms has unique uses. As it gets harder every day to understand the information we are receiving, our first step is learning to gather relevant data and—more importantly—to understand it.
Learn Latest Tutorials
ML focuses on the development of programs so that it can access data to use it for itself. The entire process makes observations on data to identify the possible patterns being formed and make better future decisions as per the examples provided to them. The major aim of ML is to allow the systems to learn by themselves through experience without any kind of human intervention or assistance.
- To ensure speedy deliveries, supply chain managers and analysts are increasingly turning to AI-enhanced digital supply chains capable of tracking shipments, forecasting delays, and problem-solving on the fly.
- “ZenML is sort of the thing that brings everything together into one single unified experience — it’s multi-vendor, multi-cloud,” ZenML CTO Hamza Tahir said.
- The system learns to recognize patterns and make valuable predictions.
- A Machine Learning Engineer must have a strong background in computer science, mathematics, and statistics, as well as experience in developing ML algorithms and solutions.
Theory of mind is the first of the two more advanced and (currently) theoretical types of AI that we haven’t yet achieved. At this level, AIs would begin to understand human thoughts and emotions, and start to interact with us in a meaningful way. Here, the relationship between human and AI becomes reciprocal, rather than the simple one-way relationship humans have with various less advanced AIs now. Fueled by the massive amount of research by companies, universities and governments around the globe, machine learning is a rapidly moving target.
Trending Technologies
One is allowing people to ask questions about designing societies—both utopian and dystopian views are formed. Where those creations have been the topics of novels for a while, the questions the books have posed are, today, reality. In a sense, people are freed from having to align their purpose with the company’s mission and can set out on a path of their own—one filled with curiosity, discovery, and their own values.
- An ML model exposed to new data continuously learns, adapts and develops on its own.
- In healthcare, machine learning is used to diagnose and suggest treatment plans.
- Since the main objective of AI processes is to teach machines from experience, feeding the correct information and self-correction is crucial.
- Deep learning algorithms are essentially self-training, in that they’re able to analyze their own predictions and results to evaluate and adjust their accuracy over time.
Practitioners in the AI field develop intelligent systems that can perform various complex tasks like a human. On the other hand, ML researchers will spend time teaching machines to accomplish a specific job and outputs. The term artificial intelligence was first used in 1956, at a computer science conference in Dartmouth. AI described an attempt to model how the human brain works and, based on this knowledge, create more advanced computers. The scientists expected that to understand how the human mind works and digitalize it shouldn’t take too long.
types of machine learning algorithms
Deep learning essentially means that, when exposed to different situations or patterns of data, these algorithms adapt. That’s right, they can adapt on their own, uncovering features in data that we never specifically programmed them to find, and therefore we say they learn on their own. This behavior is what people are often describing when they talk about AI these days. Today, AI is a term being applied broadly in the technology world to describe solutions that can learn on their own. These algorithms are capable of looking at vast amounts of data and finding trends in it, trends that unveil insights, insights that would be extremely hard for a human to find. They are trained to perform very specialized tasks, whereas the human brain is a pretty generic thinking system.
Business requirements, technology capabilities and real-world data change in unexpected ways, potentially giving rise to new demands and requirements. As this is a developing field, terms are popping in and out of existence all the time and the barriers between the different areas of AI are still quite permeable. As the technology becomes more widespread and more mature, these definitions will likely also become more concrete and well known. On the other hand, if we develop generalised AI, all these definitions may suddenly cease to be relevant.
· Types of algorithms
Unsupervised machine learning algorithms don’t require data to be labeled. They sift through unlabeled data to look for patterns that can be used to group data points into subsets. Most types of deep learning, including neural networks, are unsupervised algorithms. Explaining how a specific ML model works can be challenging when the model is complex. In some vertical industries, data scientists must use simple machine learning models because it’s important for the business to explain how every decision was made. That’s especially true in industries that have heavy compliance burdens, such as banking and insurance.
ML models can only reach a predetermined outcome, but AI focuses more on creating an intelligent system to accomplish more than just one result. Another difference between AI and ML solutions is that AI aims to increase the chances of success, whereas ML seeks to boost accuracy and identify patterns. Because AI and ML thrive on data, ensuring its quality is a top priority for many companies. For example, if an ML model receives poor-quality information, the outputs will reflect that. ML is a subset of AI, which essentially means it is an advanced technique for realizing it.
Business
However, firstly, machine learning access a huge amount of data using data pre-processing. This data can be either structured, semi-structured, or unstructured. Further, this data is fed through some techniques and algorithms to machines, and then based on previous trends; it predicts the outputs automatically.
AI will see a widespread application in mundane and process-oriented tasks such as accounts payable, loan underwriting, software engineering, and even radiology. Artificial Intelligence and Machine Learning have made their space in lots of applications. Even businesses are able to achieve their goal efficiently using them. And the most important point is that the amount of data generated today is very difficult to be handled using traditional ways, but they can be easily handled and explored using AI and ML.
Read more about https://www.metadialog.com/ here.